Key Takeaways for Leaders
Estimation isn’t enough: Traditional estimates fail in dynamic environments, leading to missed deadlines and broken trust.
Flow metrics reveal reality: They provide insights into how work flows through your system, encompassing both active and wait times.
Monte Carlo simulations boost confidence: They use your historical data to forecast outcomes and risks, replacing guesswork with probabilities.
Adaptability is key: Re-running forecasts when conditions change allows you to plan proactively and communicate progress confidently.
Scale effortlessly: Flow metrics and Monte Carlo work at every level, from individual teams to portfolios, giving you clarity across the organisation.
Introduction: Why Traditional Estimation Fails You
Think back to the last time your team promised to deliver a project by a specific date. How close did you come? Chances are, even if you hit the deadline, it came at a cost — extra hours, weekend work, stressed teams, and probably a final product that wasn’t quite what you’d envisioned. If you missed the deadline, the fallout was probably worse: frustrated stakeholders, eroded trust, damaged team relationships, followed by a frantic scramble to reset expectations.
It’s not your team’s fault. The problem lies in the process — traditional estimation masquerading as certainty. For decades, we’ve relied on detailed guesses, dressed up as plans, to predict the future. But the reality is that our businesses are too complex, our systems too dynamic, the markets we operate in are in constant flux, and our work too variable for estimation to give us anything more than a false sense of certainty.
“It ain’t what you don’t know that gets you into trouble. It’s what you know for sure that just ain’t so.” — Mark Twain (attributed)
What if there were a better way? A way to plan and forecast that doesn’t rely on guesswork but instead uses data — data generated by your teams’ process — to show the range of what’s possible at different levels of confidence based on your risk tolerance. In a world where decisions must be made quickly and confidently, data — not guesswork — is the only reliable foundation. That’s precisely what flow metrics and Monte Carlo simulations offer. They replace the anxiety of “Will we/won’t we make it?” with the confidence of “Given what we know now, here’s what’s likely.”
In this post, I’ll show you why flow metrics and Monte Carlo simulations are game-changers for senior leaders like you. We’ll explore why they work, how they’re better than traditional estimation, and, most importantly, how you can start using them today to make better, data-driven decisions.
Why Leaders Struggle with Estimates (And How to Fix It)
Let’s be honest: traditional estimation feels like the right thing to do. After all, how can you plan a project without knowing how long it will take or how much it will cost? The problem is that estimates are often little more than educated guesses — and the more complex the work, the less likely those guesses align with reality!
Here’s why estimation fails time and again:
1. The Flaw of Averages
Most estimates boil down to averages, and averages hide the truth. When you estimate that a project will take six weeks, what you’re really saying is, ‘It might take four weeks if we’re lucky or ten weeks if things go sideways.’ But that range is rarely communicated, and decisions are made as though six weeks is guaranteed. What’s worse, the average often represents only a 50% likelihood — like flipping a coin and hoping for heads. The result? Missed deadlines and broken trust.
“Plans based on average assumptions are wrong on average.” — Dr Sam Savage (The Flaw of Averages: Why We Underestimate Risk in the Face of Uncertainty)
2. Cognitive Biases
Human brains are hardwired for optimism and overconfidence. We underestimate how long tasks will take, overestimate our capacity to deliver, and anchor ourselves to the first number someone throws out in a planning meeting that, based on our experience, sounds good. These biases make estimation inherently unreliable, no matter how much experience we think we’re bringing to the task.
3. The Effort vs. Return Paradox
Teams can spend hours — sometimes days — debating and refining estimates, trying to predict the unpredictable. And what do they get for their trouble? A number that still turns out to be wrong half the time. That time and effort could have been spent delivering value instead of arguing over guesswork.
4. The Problem of Active Time
When people or teams estimate how long a task will take, they usually focus on ‘active time’ — the time they’ll spend directly working on the task. What they often overlook is waiting time: delays caused by dependencies, competing priorities, or unforeseen blockers, whether internal or external to the team. These delays can account for the majority of a task’s total time in the system. The result? Estimates based on active time tend to be overly optimistic, leading to unrealistic expectations for delivery.
5. The “One and Done” Mentality
I’ve talked about this mentality before in another blog about treating experimentation as a one-and-done. Here, I’m addressing the same issue with estimates — treating them as static while the real work isn’t. Priorities change, understanding of the work evolves (often revealing the need for more effort), and unexpected blockers appear. Yet estimates rarely adapt to these realities, leaving teams stuck chasing a plan that no longer makes sense.
6. Estimation as a False Security Blanket
Estimates make us feel like we’re in control. But that control is an illusion. Planning based on estimates often gives leaders a false sense of certainty, leading to overly rigid plans that can’t adapt to the inevitable variability of actual work. Under pressure to stick to the plan, teams often work furiously to deliver right up to the end, reporting progress as ‘green’ even when it’s anything but — a phenomenon we often call a ‘watermelon project’: green on the outside but red in the middle.
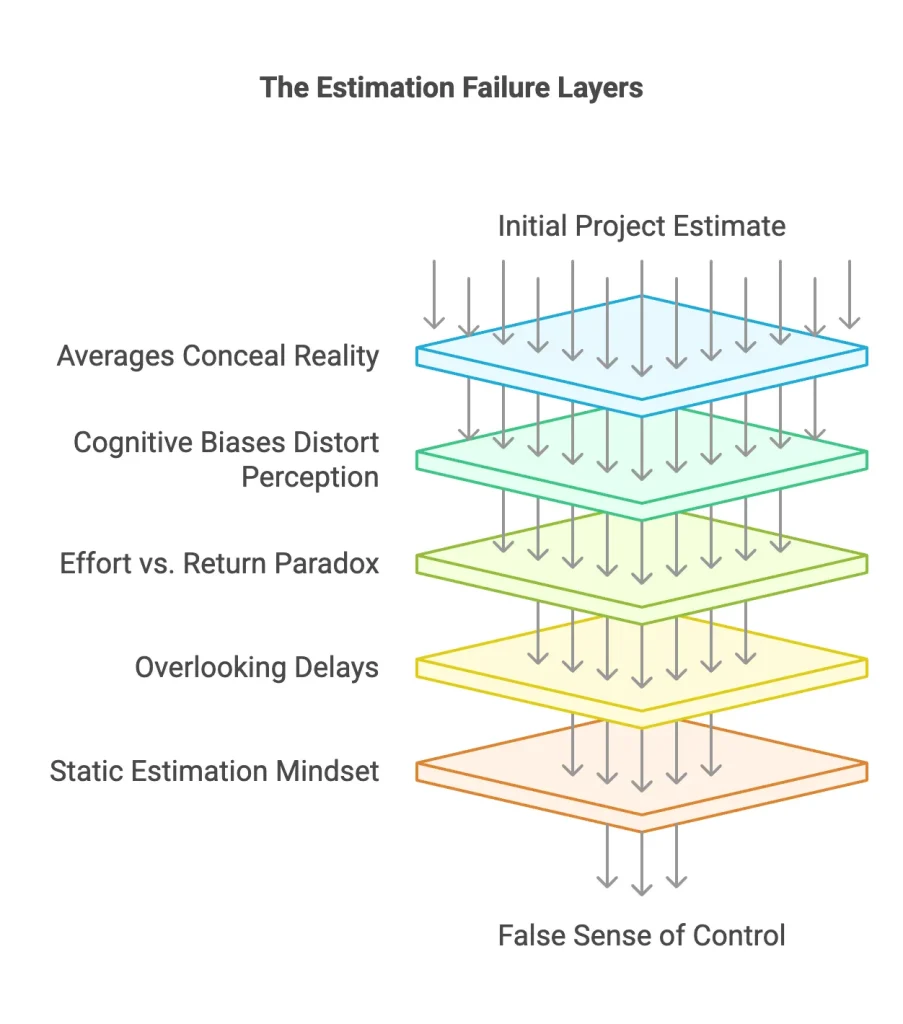
The Bottom Line: Estimation doesn’t fail because people are bad at it — it fails because it’s the wrong tool for the job. Complex, dynamic systems demand a different approach that accounts for variability, adapts to change, and gives you actionable insights instead of false promises.
Fortunately, that approach exists. It starts with flow metrics and Monte Carlo simulations, and let’s talk about that next.
From Guesswork to Clarity: Why Leaders Need Flow Metrics
If estimation is guesswork, flow metrics are reality. They don’t rely on assumptions about the future — they’re based on data from your past performance. Instead of trying to predict outcomes with precision (and usually failing), flow metrics focus on patterns and probabilities, giving you a clearer, more actionable view of your system.
At their core, flow metrics help you understand how work moves through your system. There are four key metrics you need to know:
1. Throughput
Throughput is the number of work items your team completes in a given time period. Think of it as your delivery speed. Instead of asking, ‘How long will this take?’ throughput helps you ask, ‘How much can we finish in the next four weeks based on what we’ve done before?’ This approach assumes that the future will resemble the past — an assumption that holds when your system and process remain stable. Recognising and maintaining that stability is key to making throughput a reliable forecasting tool.
2. Cycle Time
Cycle time measures how long it takes to complete a single work item — from start to finish. It’s a critical metric for understanding how long items typically flow through your system and identifying bottlenecks. To make this metric meaningful, you and your team need to agree on what ‘started’ and ‘finished’ mean for your workflow, as these points can vary between teams or even between types of work. If cycle times are increasing, something is slowing work down, and flow metrics give you the insight to pinpoint and address the cause.
3. Work in Progress (WIP)
WIP tracks the number of work items currently in progress. Why does this matter? Because the more work you start, on average, the longer everything takes, on average. WIP is a key lever for maintaining flow, helping teams avoid overloading the system and creating bottlenecks.
4. Work Item Age
Work item age is the silent hero of flow metrics. It tells you how long a work item has been in progress, giving you an early warning system for delays. If an item’s age creeps up, it’s time to ask: “What’s slowing this down?”
Why Flow Metrics Work
Flow metrics are powerful because they’re grounded in your team’s historical performance. Unlike estimates focusing solely on active time, flow metrics encompass both active and waiting times, giving you a complete picture of how work flows through your system. They adapt to variability instead of ignoring it, showing you the true dynamics of your process. Here’s a summary of why they’re better:
Data Over Guesswork: Flow metrics replace predictions with facts, giving you a more honest and comprehensive view of what’s achievable, given your risk appetite.
Visibility and Control: Flow metrics help you see where work gets stuck, how fast things are moving, and when items are taking too long. By actively monitoring the flow rather than passively observing the end results, you can proactively address bottlenecks and keep cycle times predictable.
Scalable Insights: Whether you’re managing a single team or a portfolio of projects, flow metrics scale effortlessly to provide valuable insights at every level.
Example: How Flow Metrics Shift the Conversation
Imagine a leadership meeting. Instead of arguing whether the next project will take 6 weeks or 10, you’re looking at a throughput chart. It shows your team typically completes 5–7 work items per week. This range is based on historical averages, reflecting the team’s usual performance rather than a single guess. You can use this data to say:
“Based on our current throughput, we can reasonably expect to deliver 30–35 work items in six weeks. Let’s prioritise what’s most important in that range.”
This isn’t guesswork. While the forecast uses averages, it’s grounded in real data, making it far more reliable than traditional estimates. Flow metrics help you respect your team’s capacity and focus on delivering value, not chasing arbitrary deadlines.
Flow metrics don’t just change how you plan — they change how you lead. And when you combine them with the power of Monte Carlo simulations, you take the next step in data-driven decision-making by incorporating risk.
Planning for Confidence: Why Monte Carlo Works for Leaders
If flow metrics show you where you are, Monte Carlo simulations show where you will likely go. Together, they take the guesswork out of planning and replace it with data-driven confidence.
Monte Carlo simulation might sound intimidating, but it’s surprisingly simple in practice. Here’s how it works:
1. Start with Data: Use your team’s historical throughput (how many work items you typically complete in a given time period, usually daily).
2. Run Simulations: The simulation runs thousands of scenarios based on this data, accounting for the natural variability in your system.
3. Get a Range of Outcomes: Instead of a single “estimate,” you get a range of possibilities and their likelihoods — for example, an 85% chance of completing 50 items in 10 weeks.
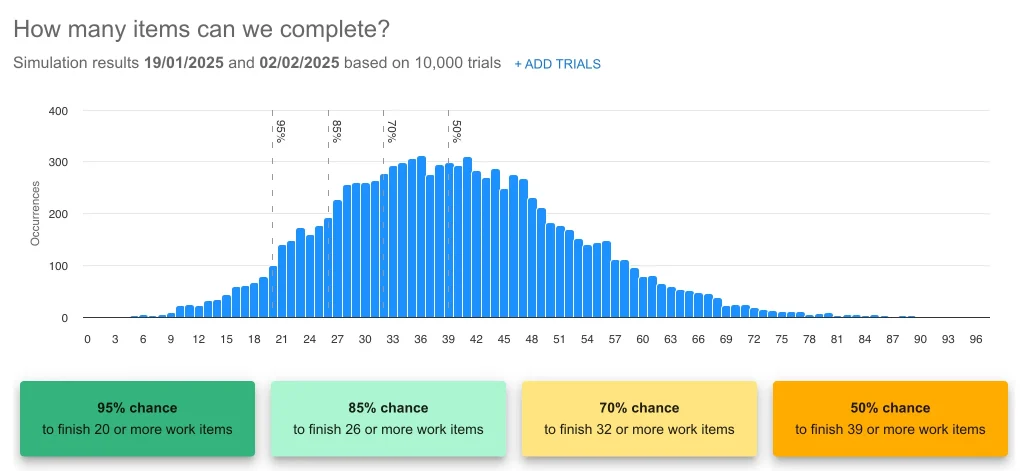
Why Stability Matters
Monte Carlo simulations are powerful tools but rely on stable processes to deliver meaningful insights. If your team’s workflow is inconsistent — frequent priority shifts, irregular throughput, or unpredictable bottlenecks — your forecasts may reflect that instability, leading to misleading results.
To ensure reliable forecasts, assess your process stability before using Monte Carlo. Tools like Process Behavior Charts (PBCs) can help identify patterns in your system and highlight areas of variability. Stabilising your process doesn’t just improve forecast accuracy — it builds trust and confidence in data-driven decision-making, making it easier to counter scepticism about moving beyond traditional estimation.
What Makes Monte Carlo Different?
Monte Carlo simulations give you a probabilistic forecast rather than a deterministic guess. This is a big deal, and here’s why:
1. Probabilistic Confidence
Instead of saying, “We’ll definitely finish in six weeks,” you can say, “We have an 85% chance of finishing in six weeks and a 95% chance of finishing in seven.” This confidence range is far more helpful for decision-making because it reflects reality — things don’t always go perfectly, and that’s okay.
2. Adaptability to Change
Monte Carlo simulations don’t freeze your plan in time. They adapt as your team’s throughput changes. If your delivery pace slows due to unexpected blockers, the simulation reflects that when it is next run, helping you adjust expectations and priorities in real-time.
3. Risk Management, Simplified
Monte Carlo simulations make risks visible. For instance, if your forecast shows a low probability of hitting a critical deadline, you can address the risk early — reprioritise, allocate more resources and/or people, or adjust scope — before it becomes a crisis.
4. Speed and Simplicity
You don’t need weeks of data science to run a Monte Carlo simulation. Most tools can generate results in seconds, and you only need a few weeks (sometimes days) of throughput data to get started.
5. Real-World Scenario Planning
Monte Carlo simulations allow you to run “what-if” scenarios to model different outcomes based on varying conditions. You can adjust throughput periods to account for differing rates of progress, scale throughput to accommodate holidays or reduced team availability, and explore how these factors impact delivery timelines. This flexibility gives you powerful, real-world modelling capabilities, enabling you to plan more effectively and make informed decisions in dynamic environments.
From Burnup to Breakthrough: Making Data Work for You
Many leaders are familiar with burnup charts, which track cumulative progress over time. While helpful for visualising completed work, they often fall short in dynamic environments where priorities shift and uncertainty reigns.
Monte Carlo simulations build on this by factoring in variability and giving you probabilities rather than fixed predictions. Here’s the difference:
Burnup Chart: Shows historical progress and projects future work based on linear assumptions.
Monte Carlo Simulation: Models variability to provide confidence intervals, enabling you to plan for uncertainty and communicate risks effectively.
Imagine using Monte Carlo to augment your burnup chart. Instead of hoping the trend line holds, you can say:
“There’s a 75% chance we’ll finish by the end of March, but if scope creeps or team availability drops, we have fallback plans ready.”
This shift from static to dynamic forecasting gives you the flexibility to adapt and manage expectations confidently.
A Simple Analogy: The Weather Forecast
Think of Monte Carlo like a weather app. It doesn’t tell you the exact temperature at 3:00 PM next Thursday, but it does tell you the range of likely temperatures and that there’s an 80% chance of rain. Armed with that information, you should definitely bring an umbrella and maybe a coat!
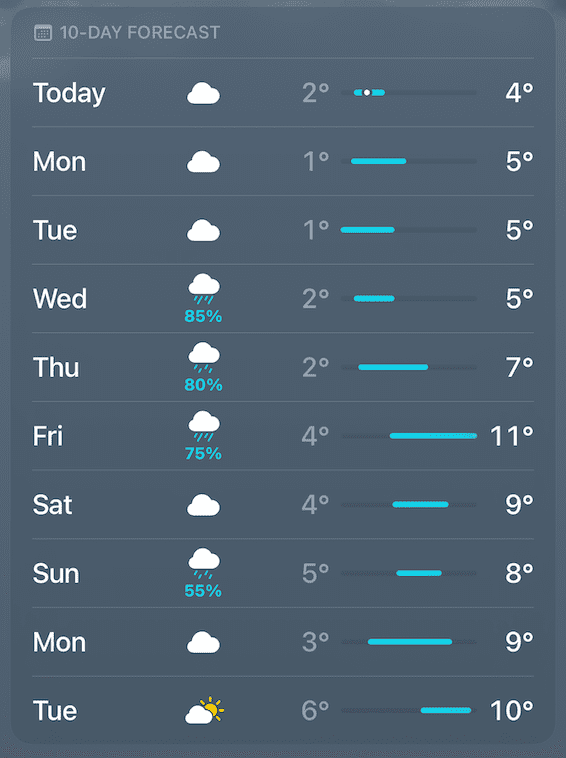
Monte Carlo does the same for your projects. It doesn’t promise you’ll finish on an exact date, but it shows the likelihood of various outcomes, helping you prepare and plan effectively.
Real-World Example: Planning with Monte Carlo
Imagine you need to deliver 100 work items for a major product launch. With Monte Carlo, you can ask:
“How likely are we to finish all 100 items in 6 weeks?” Answer — a little over 70%.
“What’s the 85th percentile date for completing 80 items?” Answer — 23rd February.
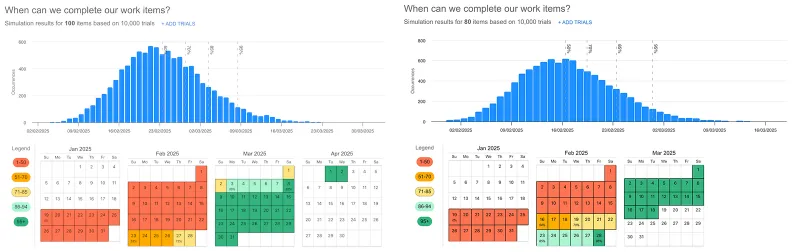
The simulation might reveal that completing all 100 in 6 weeks has only a 70% probability, but completing 80 items has an 85% probability. Armed with this insight, you can confidently adjust scope and priorities, delivering value without overpromising.
The Bottom Line
Monte Carlo simulations turn uncertainty into actionable insights. They empower you to make informed decisions, manage risks, and set realistic expectations with your stakeholders.
Combined with flow metrics, Monte Carlo simulations are the next step for leaders who want to move beyond gut feelings and static estimates. They give you clarity, control, and confidence in your delivery process.
And the best part? You don’t need to be a mathematician to use them. You simply need the right mindset and a willingness to let data guide your decisions.
Why Flow Metrics + Monte Carlo > Estimation
Flow metrics and Monte Carlo simulations aren’t just ‘better’ than traditional estimation — they fundamentally change how you approach planning and decision-making. Rather than relying on best guesses and static timelines, they provide data-driven insights that adapt to your team’s evolving reality. Here’s what your new status quo looks like with Monte Carlo simulations:
1. Predictions Become More Reliable as Data Reflects Reality
Estimates are static snapshots, often made without enough information and quickly rendered obsolete. Flow metrics and Monte Carlo simulations, on the other hand, become more effective as you actively use the data to refine your understanding and decision-making.
Why it matters: Your team’s throughput, cycle times, and variability are baked into the forecast, meaning that as you continue to measure and incorporate this data into your process, your predictions become more reliable and actionable.
2. Adaptive Planning for Dynamic Work Environments
Traditional estimates can’t flex when priorities shift, or unexpected blockers arise. However, flow metrics and Monte Carlo simulations are dynamic — they reflect your current system state every time you re-run the forecast.
Example: Imagine your throughput slows due to an unplanned event or a key assumption — like team availability — proves to be incorrect. By re-running the Monte Carlo simulation with updated data, you generate a revised delivery forecast that aligns with your new reality. This iterative approach to forecasting provides actionable insights, enabling you to adjust priorities, plan proactively, and communicate progress confidently rather than scrambling to react after the fact.
3. Focus on What’s Likely, Not What’s Perfect
Estimates often set unrealistic expectations because they focus on a single point in time — usually the “best case.” Flow metrics and Monte Carlo embrace variability, helping you plan for a range of outcomes.
Why it matters: Instead of overpromising and underdelivering, you can confidently say, “We’re 85% sure we’ll finish on or before this date,” building trust with stakeholders.
4. Drive Better Conversations
Flow metrics and Monte Carlo simulations shift the conversation from “How long will this take?” to “What does our data tell us is possible, given our risk appetite?” This removes subjectivity and guesswork, leading to more productive discussions.
Bonus: When data back decisions, aligning teams and stakeholders around realistic goals is more straightforward.
5. Manage Risk Proactively
With traditional estimates, risks often surface too late — when deadlines are missed. Flow metrics and Monte Carlo simulations make risks visible upfront, allowing you to address them early.
Example: If a forecast shows low confidence in hitting a key date, you can adjust priorities, allocate resources/people, or communicate updated expectations proactively.
6. Build Trust Across the Organisation
Nothing erodes trust like missed deadlines or unkept promises. By using flow metrics and Monte Carlo, you’re improving accuracy and fostering transparency.
Why it matters: Leaders like you, teams, and stakeholders feel confident in the process because decisions are grounded in data, not gut feelings.
7. How Leaders Can Scale Predictability Across Teams and Portfolios
Estimates become more challenging to manage as work scales — more teams, projects, and dependencies create additional complexity. Flow metrics and Monte Carlo simulations thrive in these environments, offering insights for anything from individual stories to entire portfolios.
Why it matters: Monte Carlo approaches can be applied to higher-level work, such as epics, features, or entire portfolios, helping you forecast delivery timelines and manage risks across multiple work streams. This scalability ensures you can make informed, data-driven decisions at every level, from team backlogs to strategic initiatives, without adding unnecessary overhead.
The Big Picture
Flow metrics and Monte Carlo simulations aren’t just tools but a mindset shift. They empower you to plan based on reality, adapt to change, and confidently manage uncertainty.
By embracing these approaches, you’re not just improving delivery outcomes — you’re transforming how your organisation thinks about planning, risk, and success.
Traditional estimation served its purpose in a simpler, more predictable world. Today’s fast-paced, dynamic environment demands something better. Flow metrics and Monte Carlo simulations are the tools that will take you there — it’s time to leave the guesswork behind.
“For leaders, the value of flow metrics and Monte Carlo lies in their ability to align teams, foster transparency, and consistently deliver value while mitigating risks. By adopting these tools, you set your organisation up for long-term, scalable success.”
Actionable Steps to Get Started
Implementing flow metrics and Monte Carlo simulations doesn’t have to be overwhelming. You can start small and see immediate benefits. Here’s how to take your first steps:
Step 1: Start Measuring Flow
To use flow metrics, you need data. Begin by tracking throughput, cycle time, work in progress (WIP), and work item age. Tools like Kanban boards or workflow management software (e.g., Jira, Trello) can help you effortlessly collect this data and make it visible to your team.
Step 2: Visualise Your System
Make work visible so you can identify patterns and bottlenecks:
Use a Kanban board to map your workflow.
Track and actively manage ageing items to address delays before they become critical.
To drive transparency and collaboration, put these visuals front and centre with your team and review them regularly.
Step 3: Run a Monte Carlo Simulation
With just a few weeks of throughput data (possibly days), you can start running simulations:
Use tools like Excel, Monte Carlo-specific apps like ActionableAgile, or platforms that integrate with your workflow system.
Simulate thousands of possible scenarios to forecast your work's completion.
Focus on confidence intervals — e.g., “We’re 85% confident we’ll finish on or before this date.”
Step 4: Compare to Your Current Estimates
Run an experiment: compare a Monte Carlo forecast to your team’s traditional estimate for the same project or milestone.
Look at how close the actual outcome is to each prediction.
Use this comparison to build confidence in the data-driven approach.
Step 5: Shift the Conversation
Encourage your team and stakeholders to focus on probabilities and ranges instead of single-point deadlines:
Reframe planning discussions around what’s likely and what risks need addressing.
Use your flow metrics and Monte Carlo results to prioritise and allocate resources and people.
Step 6: Improve as You Go
Flow metrics and Monte Carlo are not just about forecasting — they’re tools for continuous improvement:
Identify bottlenecks: Use cycle time, WIP data and Work Item Age to find and fix the slow points in your system.
Refine your inputs: The cleaner and more reflective your data is of the team’s reality, the better your forecasts will be.
Celebrate predictability: Share wins with your team when forecasts align with reality — it builds trust in the process.
“Without data, you’re just another person with an opinion.”— W. Edwards Deming
Step 7: Scale Across the Organisation
Once you’ve seen success at the team level, expand the approach to other areas:
Aggregate data across multiple teams to improve portfolio-level forecasting.
Use flow metrics to make strategic decisions about capacity and the allocation of resources and people.
Embed the practice into your organisation’s planning culture.
In Closing: A New Way of Thinking
For years, traditional estimation has been the go-to approach for planning. But in today’s dynamic, complex environments, it’s clear that guesswork disguised as certainty isn’t enough. Flow metrics and Monte Carlo simulations offer a better way that’s grounded in data, adapts to change, and gives you the confidence to make informed decisions.
This isn’t just about improving forecasts — it’s about changing how we think about work. It’s about acknowledging that uncertainty isn’t a problem to be solved but a reality to be managed. With flow metrics and Monte Carlo, you don’t have to gamble on “perfect predictions”. Instead, you use probabilities to manage risk, align expectations, and deliver value with greater predictability.
It’s time to move beyond guesswork and bring clarity, confidence, and adaptability to your planning process. The tools are ready. Are you?